40. Profitable sustainability with AI- all just Buzzwords?: Interview with Ferolabs co-founder Berk Birand
- maria02715
- Feb 6
- 45 min read
Updated: Mar 27
In a world increasingly aware of climate urgency, how can artificial intelligence become a practical tool for sustainability—not just a buzzword?
In this episode, Berk Birand, co-founder and CEO of Fero Labs, tells us about their vision, where AI doesn’t just optimize processes—it reshapes entire industries.
Berk and his co founding (Alp Kucukelbir & Pamir Ozbay) teams focus is on bridging academia and industry, overcoming skepticism toward AI, and promoting profitable sustainability. He has encountered challenges such as resistance to AI adoption and early customer skepticism. Key market trends show industrial AI adoption is low but projected to grow significantly.
While Fero Labs has carved out a strong position in industrial AI, it operates in a space with well-known hurdles. Across the manufacturing sector, companies face persistent challenges such as inconsistent data quality, fragmented systems, and legacy infrastructure that wasn't built with AI in mind. Engineering teams are often overextended, making it difficult to adopt new tools without disrupting operations. On top of that, there's a growing pressure to meet sustainability goals and compliance standards—all while maintaining profitability. These aren’t problems unique to Fero Labs, but they define the environment any AI solution must succeed within.
You can listen to this episode here:
You can also listen to this podcast in Apple Podcast, amazon music or wherever you get your podcasts:

From Teen Coder to Industrial Trailblazer Berk Birand’s story begins in Turkey, where his early fascination with computers and circuits laid the groundwork for a life in tech. After pursuing dual degrees in electrical engineering and computer science in the U.S., he continued to Columbia University for a PhD focused on network optimization—a crossroads of engineering, software, and applied mathematics.
But academia wasn’t the final stop.
During his doctoral research, Birand began exploring the practical impact of software in real-world systems. Collaborating with peers working on fiber optic networks, he discovered the transformative potential of bringing AI into the physical world.
The idea? Use machine learning to optimize not just digital systems, but massive industrial machines—factories, furnaces, and chemical plants.
Enter Fero Labs: AI for Sustainable Industry
Fero Labs unique selling points include explainable AI, real-time optimization, and sustainability integration. They have strategic partnerships with Microsoft and Siemens, and collaborations with research organizations.
In Berk's words, "20 years ago, sustainability would not be one of the parameters that would affect company goals. There would be goals around cost cutting, profitability yields, or maximizing the top line and quality. And it would try to find that middle ground that satisfies all of these in terms of the costs. Now there are additional goals and regulations around sustainability. That makes things even more complex than before. So now engineers have to deal with all the same problems plus the how do we combine the manufacture, the sustainability angle."
In 2015, that idea became Fero Labs, a software company aimed at reducing waste and improving efficiency in manufacturing. From the beginning, Fero Labs targeted sectors like steel, chemicals, and cement—industries that are both highly complex and deeply impactful on the environment.
Rather than acting as a mysterious “black box,” their platform analyzes thousands of variables and tells engineers what went wrong, why, and how to fix it. The software even suggests how to tweak future batches, minimizing energy usage, material waste, and costly rework.
The result is what Birand calls “profitable sustainability”—a rare alignment between economic and environmental goals.
“Factories worldwide can reduce 800 megatons of emissions just by making slightly better decisions using AI,” he says. “That’s not hypothetical—it’s measurable.”
How is Fero Labs contributing to solving the Climate Crisis
Industrial operations, especially in steel, cement, and chemicals, are among the world’s largest emitters of greenhouse gases. By leveraging AI to reduce energy consumption, cut raw material waste, and optimize production schedules, Fero helps companies hit their climate targets without sacrificing performance.
With labor shortages on the rise and expert knowledge retiring out of the workforce, AI can assist human engineers, not replace them. The result is smarter, faster decision-making with a human in the loop.
🧠 Black Box vs. White Box AI: What's the Difference?
🔒 Black Box AI
You give it data and it gives you an answer — but you don’t know how it got there.
It’s often accurate but lacks transparency.
Common in image recognition, voice assistants, or complex deep learning systems.
➡️ Example: A black box AI says “add more carbon to the steel mix” but doesn’t explain why.
🔎 White Box AI
Also known as explainable AI (XAI).
Offers predictions plus the reasoning behind them.
You can see which data points influenced the decision and how confident the model is.
➡️ Example: A white box AI not only recommends adding more carbon but tells you it’s due to the chemistry of the current steel scrap and specific rolling mill conditions.
👷 Why Engineers Need White Box AI
In industries like steel, cement, and chemicals, decisions are high-stakes. Mistakes cost millions, damage equipment, and can increase emissions. There are the main benefits of a model such as Faro Labs proposes:
Trust & Adoption: Engineers won’t trust software that acts like magic. They need to understand and verify why an AI model recommends a change.
Root Cause Analysis: Engineers are trained to solve problems by finding the root cause. Fero’s white box AI aligns perfectly with this mindset by surfacing cause-and-effect relationships.
Collaboration & Control: Instead of replacing engineers, Fero’s AI augments their decisions, helping them work faster and smarter.
Future-Proofing: When senior experts retire, white box AI helps capture institutional knowledge and ensures continuity for the next generation of engineers.
🌍 How This Helps the Climate Crisis
Manufacturing is responsible for around 30% of global CO₂ emissions. The path to reducing this is not just building new factories—it’s making existing ones more efficient.
Fero Labs’ white box AI contributes by:
✅ Reducing Emissions
Finds inefficiencies and emissions drivers that humans might miss.
Optimizes for both cost and carbon — even adapting to Scope 1, 2, and 3 reporting.
✅ Accelerating Change
Because engineers trust and understand the system, they’re more likely to adopt sustainable practices.
Changes that would normally take months of trial and error can be implemented confidently in days.
✅ Minimizing Waste
Helps avoid production defects and scrap, which means less wasted material and energy.
In this episode we address the following questions:
[00:17]❓ “If someone were to describe you at a dinner party, what’s the funniest or most unexpected thing they’d say?”
[01:24]❓ “Can you explain what you studied and how it led you to where you are now?”
[04:31]❓ “Tell us about your journey — where did you grow up, and how did you go from a PhD to founding Fero Labs?”
[09:30]❓ “So Fero Labs was your first real company job — what was that like?”
[12:31]❓ “When you started in 2015, what vision did you have in mind?”
[15:13]❓ “Can you walk us through a typical project? What’s the challenge and how do you solve it?”
[22:21]❓ “And how does sustainability play a part in all this?”
[25:15]❓ “Looking back, how has the perception of AI and sustainability changed over the years?”
[32:41]❓ “What’s your take on the labor shortage vs. ‘AI stealing jobs’ debate?”
[38:06]❓ “You mentioned building your own models — can you explain how they’re different from LLMs?”
[42:05]❓ “Have you ever had an engineer go from distrusting AI to fully embracing it?”
[46:00]❓ “What excited investors more — the AI or the sustainability angle?”
[50:49]❓ “How are things feeling right now for German manufacturing? Are you optimistic?”
[52:14]❓ “Looking back over 10 years, was there a tough moment that taught you something crucial?”
Memorable quotes from the episode by Berk:
[03:00]🗣️ “The amazing thing about software is that you can sit in your room, create something, and someone across the world can find value in it.”
[07:30]🗣️ “The intersection between software intelligence and the physical world — that’s where the real potential opened up for me.”
[13:00]🗣️ “Profitable sustainability is what we do — improving profitability and reducing emissions at the same time.”
[18:00]🗣️ “What would take engineers weeks to figure out, AI can do in seconds with the right data.”
[24:50]🗣️ “We now optimize not only for financial cost but also for environmental cost — and let clients choose their priority.”
[30:22]🗣️ “I'm hopeful, not because humanity will magically hold hands, but because science and technology are already pushing us forward.”
[42:24]🗣️ “Engineers don’t want a black box. They want to understand why the AI is making a recommendation.”
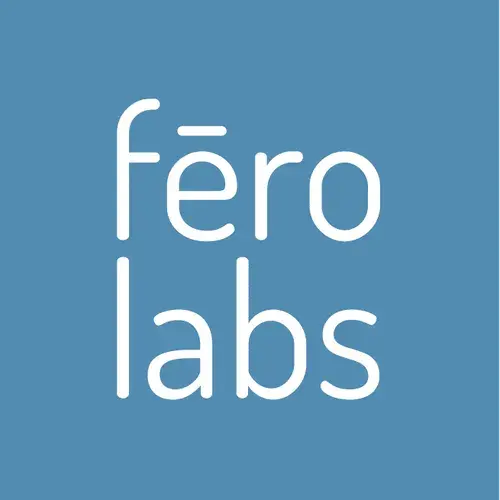
Website: https://www.ferolabs.com/
Contact Berk: https://www.linkedin.com/in/berkbirand/
__________________________________________________________________________________
Transcript based on AI and beta- status:
Friederike von Waldenfels (00:01.991)
Berg, thank you so much for joining my podcast today. I'm very excited to talk about something very relevant right now. AI and sustainability. What a mix.
Berk Birand (00:13.462)
It is an interesting mix and it's definitely an interesting time to talk about this mix.
Friederike von Waldenfels (00:17.063)
So before we dive into AI and sustainability, let's break the ice a little bit. I just said it before, it's not so easy to find something about you personally. So I thought I'm going to spice it a little bit up at the beginning. So if someone were to describe you at a dinner party, what's the funniest and most unexpected thing they would say about you?
Berk Birand (00:42.382)
I think the most unexpected thing would be in a dinner party context. I've gotten really deep into cooking stews and curries in the past few years. And I've discovered or rediscovered the electric pressure cooker. And I've gone deep off the deep end. So I love making curries from across the world, from India to Thailand. And yeah, I think it's
Potentially the dinner party is one in which I am serving one of those curries.
Friederike von Waldenfels (01:16.081)
So you're putting a lot of pressure to pressure cooking.
Berk Birand (01:19.406)
Exactly. A lot of pressure and then release all the pressure and the outcome is fantastic.
Friederike von Waldenfels (01:24.967)
I love this. So were you like this already? What did you study exactly? I tried to get the right name on that. Can you explain a little bit what you exactly studied?
Berk Birand (01:40.034)
Yeah, my PhD was in the electrical engineering and computer science area. So officially in the EE department, but I did research in theoretical parts of electrical engineering. So it wasn't this weird intersection where electrical engineers wouldn't consider me an electrical engineer because I didn't work with bolts and circuits and, you know, resistances. Computer scientists would think I'm too practical. So I wasn't too theoretical enough. So I was kind in the middle between EE, CS and maybe applied math as the third component.
But ultimately my research was around applying some of these technologies and some of these techniques to wireless optimization and network optimization.
Friederike von Waldenfels (02:17.607)
So were you already interested like this as a kid? Like did you take apart radios or computers or were you more, you know, on the theoretical side? Were you more practical or theoretical?
Berk Birand (02:29.854)
I was more practical, definitely. I was more practical. I was definitely the kid who was playing with circuits at a young age and putting together computers when I was 12. I was, I got interested in the software side around the same time, I would say 12, 13. I started, taught myself how to program. I just really liked the idea of creating something, just me sitting in my room as a teenager creating something. And at the time, it was...
places where you could share your software. SAS wasn't really a thing, so you put it on download.com. And I could, in my little room, create something and put it on some website, and somebody from across the world could find value in it. So that was the thing that really got me really interested, which is amazing that we can do this in the software world. If I was interested in civil engineering, I couldn't build a building in my room somehow. Or electrical engineering is even more equally complex. But with software, you could just create something, which is typing on a keyboard, which was just
incredible to me.
Friederike von Waldenfels (03:28.929)
Amazing. And what did Sparks then to actually study it in the end?
Berk Birand (03:34.862)
Yeah, I think I really felt like this was a thing that like my calling, if that makes sense, you know, something that I really could contribute to the world, you know, and use my skills that I've just because I skills that I developed just because I'm interested, I was interested in them. And I really think thought that this would be something that I could bring to the world in a broader sense, including the entrepreneurship component, which of course came much later after my PhD. But I was also kind of putting two and two together was
Yeah, it was an obvious choice for me to go into software engineering and electrical engineering.
Friederike von Waldenfels (04:09.223)
I can totally relate actually when I think I was like 15, 16, I started to game and my mom always called me the seller child. And then I wanted to always to know what is happening when I press the enter. I always wanted to know what is actually happening. So, and I was also like in this middle, like I studied computer science and business administration.
Berk Birand (04:20.344)
Okay.
Berk Birand (04:26.158)
the
Friederike von Waldenfels (04:31.275)
And they always said like, you can't really program. You don't really know how business works. So I can really relate to this mix of both sides. And get me through that journey of yours. where have you been growing up? When, you know, where did you go to university? And when was the point actually, when you created a PhD and in the end actually resulted into Farrow Labs?
Like, can you walk me through a little bit that journey you did and what sparked in 2015 to found FeroLabs?
Berk Birand (05:05.664)
Absolutely. Yeah, so the first part of the journey, so I was born and raised in Turkey. so Turkish parents went to a French high school in Turkey. So throughout my high school studies, I was in the French system and then kind of wanted to change things up a bit. The normal trajectory would be to go to France to do the preparatory school and the gonzi college, what they call it. But then, I think I was one to kind of switch things around and be more applied sooner. So the US system typically get to do
cool things sooner than in the French system. So then I switched trajectories a little bit, came to the US, went to school in a city called Worcester, Massachusetts, a school called Worcester Polytechnic Institute. It's a small engineering school, double majored in electrical engineering and computer science. I did my electrical engineering work in the networking space and my computer science work in the cryptography space during my undergrad. So I did research in hardware,
random number generators, which is a key component for securing circuits. And then I knew at the time when I was doing research in these fields that actually I liked being in the academic space and I'm not ready to leave yet. I'm learning a lot. I'm interacting with wonderful people. So that was the impetus to do a PhD. So that's how I ended up at Columbia here in New York, which was also my first arrival to New York almost 16, 17 years ago. And those were fantastic years.
just being doing research with fellow PhD students, teaching. I I learned a ton. And I think I started appreciating more and more, first of all, that I didn't want to necessarily continue on the academic trajectory. At some point, did consider going into academia full time as a professor. So I did want to maybe go into practice a little bit sooner than that. And also doing research in the telecom space.
theoretical research about working with actual circuits was kind of mind opening to me. know, because we had, I was developing algorithms, let's say for fiber optic networks, and we were collaborating with folks that were building these networks. So I was going to the lab of fellow PhD students. They had set up this, you a hundred mile spool of fiber and actual lasers, and then they had set up the physical system. And I was coming in with my algorithms and trying to see how we could optimize that and then reduce the
Berk Birand (07:31.246)
problems and transmissions and so forth. And then that's the intersection between intelligence, software intelligence with actual physical world and changing something in the physical world was just the potential that opened up was really exciting to me. That we could send something in a physical world, do some computation and change something in the physical world. At the time there was this hype around the term internet of things. So this notion of the internet,
In addition to being humans downloading websites, basically devices and sensors and actuators and smart cities and cars on the highway talking to each other. So that vision was kind of forming. And that was really interesting to me. So another kind of aspect of my time at Columbia was to meet my two co-founders. And one of them was my roommate. My first two years, he was doing his master's in electrical engineering.
And then the other was towards NMI PhD, my other co-founder who was doing a postdoc at the time in data science, a data science group at Columbia. So that's kind how the whole core team came together as well. And then realized that we shared a mutual interest in solving interesting problems with technology. The IoT angle of hardware software, the machine learning AI angle, and then our third co-founder,
actually went on to work for GE and spent, know, he was smart enough to not continue for a PhD. So he spent the rest of his while I was in a lab, he was working for GE, traveling around the world and to steel factories and chemicals factories. And he would see the problems that they're facing firsthand and the inefficiencies of these giant steel plants in, you know, China and Chile and elsewhere with these massive, you know, flues coming out from them. And that was kind of
where all the three components clicked together and we found that Faro as a result around 2015.
Friederike von Waldenfels (09:30.627)
Hmm. So you actually never worked like in a company before that was your first company you were actually being employed yourself into.
Berk Birand (09:40.652)
Yeah, that's true. That's exactly right. So I had to learn a lot of things from scratch. Some things that are maybe obvious to many, I had to study them. But things like, what does HR do? just Google that. So yeah, it was the first official company. I worked on other startup ideas before during my PhD, but none that came as far as as far. There were more kind of projects of sorts. So yeah, this was the first company that I worked for and still is.
Friederike von Waldenfels (10:09.415)
Can you share one of these projects you worked on and you thought like going backwards either this was a super stupid idea or that would have been actually a great idea?
Berk Birand (10:17.208)
Ha ha.
So the joke always is that there's this kind of rite of passage type of thing for entrepreneurs that every entrepreneur has to build a restaurant app at some point in their lives. So I was there. did my dues and built my restaurant app. I really thought I had an angle. This was at the beginning of my PhD. The advantage of being an engineer, which is also a disadvantage, is that if you have an idea, you can just build it. Nothing stops you, which is kind different for if you come from the business world and you have an idea of how find someone to build it.
Of course, the disadvantage of this is that, well, you just go ahead and build it without kind of thinking very hard around, is this really a need or do people, what is the competitive landscape or is there a market for this or how does it even turn into an actual thing that exists, know, self-sustaining entity that exists. So, yeah, I did my restaurant app and it didn't work out, which was fine. I think the part, the one that I was really excited about that also didn't exactly work out, we created an arts marketplace.
for artists, painters, sculptors to send their artwork on. But one of the big problems in the art world is that clearly young artists sell their artworks for relatively cheaper prices and then ultimately these works go up in price but then the initial artist doesn't really gain from this at all. And in fact, there are galleries that bought by dozens of artworks from young artists. They keep them in a warehouse for years.
until the price goes up and the artist becomes a big name and then they start trickling it to the market. And of course, the artist doesn't get anything out of this. So we wanted to change this a little bit and create an environment, a marketplace that allowed artists to sell their work. But then if the buyer wanted to sell it again, they could sell secondhand on the same platform and artists would continue getting cuts from that permanently. So that was kind of a way that we thought would be, know, revolutionizing the art world. That didn't also get very far. And I think my takeaway from that point, I wasn't, I was the...
Berk Birand (12:13.55)
CTO of that startup. And my takeaway from that was I should focus on things that I know. I should focus on things that I understand, technology side and on the market side. And hence the going back to the more technologically kind of firmer startup, which is fair.
Friederike von Waldenfels (12:31.495)
Fantastic. love these stories that, know, entrepreneurship is never a straight arrow. It's always going a lot of testing, trying out. I think many don't talk about that there has been many failures before and testing out other things to actually find them the one which is right fit for yourself and for the market. When you started 2015 Feral Labs, under which, you know, vision,
Did you started it? what, what, what we were thinking at that time, what do I want to solve and why do we think, you know, this will be creating is the right thing.
Berk Birand (13:08.782)
Yeah, the main problem that we're going to was very similar to what we are solving today, which is to make factories more efficient. The interesting angle was frankly to it that was really mind boggling to me is first of all, know, one of our co-founders, he was kept saying that, if you have, you know, go to these plants across the world and they buy multimillion dollar worth of control systems. And then, you know, within a year, you know, they send an engineer who tunes these systems for months.
And then after six months, the plant is commissioned and then the engineer leaves. then a year later, the plant goes out of tune again. And nobody tunes it. And this becomes inefficient and runs inefficiently for years, for eight, nine, 10 years, until they buy another equipment that then you need for someone to come in or to tune it. And this was quite interesting to me that why not have a system that tunes the factory continuously and bring the software in?
that can do this intelligently. And we thought that there was really a potential here of using our background to make an impact in the real world, to make an impact from, first of all, the engineers' time and engineers' efforts and allow them to do things efficiently so that they don't have to live in a factory for six months to tune something or so that it happens just automatically. Make the...
plants life easier as well in terms of their mission, their compliance. I mean, these are giant plants and they're outputting incredible amount of emissions. And ultimately, so that was the intersection with the sustainability angle, which is of course a core part of what we do now. In fact, profitable sustainability is what we call what we do, which is the intersection of both increasing profitability and sustainability at the same time. using our background, something that we know really well.
in order to make somebody else's life, who is not an expert in what we do, and we're an expert in what they do. That was the thing that really was the spark, the curiosity of like, hey, could we do this? Could we pull this off? And yeah, so here we are now, years later.
Friederike von Waldenfels (15:13.991)
almost 10 years, you know, like, uh, think it's a 10 years anniversary. Can you maybe talk us through an example, of like a typical projects you're working on? How do you see it? Like when you get there, what are the requests you're getting to actually improve and what are you doing in the end actually improving and how does it look like?
Berk Birand (15:15.308)
Yeah, yeah, hard to believe.
Berk Birand (15:36.024)
Yeah, absolutely. So we're active in several sectors and steel is still one of our core sectors. so in steel, the kind of use cases for ferro usually revolve around reducing waste. And this is kind of an interesting angle because clearly reducing waste has been central to the industrial world for many, many years. This goes back to the Six Sigma, before that the Toyota production system, lean manufacturing.
Factories have different ways of reducing waste, including some of the simple things. If we put our parts, move our parts, spare parts from this point to that other point, then we'll have to walk less to get to those parts and that will save us time and that's one form of waste. And a lot of these types of improvements, we have nothing to contribute because we're not experts in steel. We know a lot about steel, of course, but that's not our core contribution. Where our contribution comes in,
is when companies have a lot of data, they have data that is complex, that is real world data, which means that it's not perfect. There's issues with data. There's data that's in different parts of the plant, different groups co-own it in different ways. And a lot of times, a lot of the problems that manufacturers face become effectively data problems. So something went wrong in the factory. We failed our quality. Let's say, let's give a concrete example in steel.
steel manufacturers, make steel and then they have to make sure that the strength of the steel has to be within certain bounds, certain specifications. Let's say this is construction grade steel, of course it has to match the strength properties. And let's say they failed, so they spent a lot of energy, a lot of time, a lot of resources building this giant, multi-hundred ton batch of steel and it just didn't work.
Strength is wrong. Strength is off. What do they do? All right, well, let's figure out why. Let's find the data of this particular batch. Let's try to compare it with that previous batches of the same kind. Is it because of how we pressed it? Is it because it wasn't warm enough? Is it because of the chemistry in it? Well, but this other batch had the same chemistry and it did work. Okay, so then it might not be this one. And this could take weeks, definitely days, weeks. And sometimes it just becomes so involved.
Berk Birand (18:00.514)
that one group goes, well, it's probably the other group's fault, another one goes, it's probably the other guy's fault, and then they just drop it. We won't get to the bottom of it, so let's just drop it. So these kinds of problems are really well-suited for what we do at Faro, because these are the things that AI, machine learning, perfectly qualified to solve. Just give it all the data, give it what you know, tell it what you know about the factory and how it's structured, and let it figure out what is unique about this and to understand what caused this problem.
And of course, these models can go even beyond that. Instead of trying to troubleshoot what happened before, they can even predict what's going to happen in the future or allow you to change parameters. We're making steel tomorrow. We had this failure last week. How should we change the process for the next one? Well, Farrow can tell them, here's how you do it. You add a little bit less of this ingredient and you increase your temperature a little bit more. And then we believe that this is going to lead to higher strength steel.
So lot of the, I think to answer your question, a lot of the use cases revolve around digging into data and understanding and improving different efficiency metrics revolving around yield, quality, throughput and emissions. And typically, in fact, typically it's all of these at the same time. Because usually people, factories don't have a single goal. You have different teams, know, they have a quality team. Their goal is to make sure the quality is high enough. They have the maintenance team. They want to make sure that the
the equipment lasts as long as possible. So a lot of times these are aligned, but sometimes not aligned. Sometimes a decision that improves the quality is a thing that reduces the timeline of the equipment. And that's where you have to find a sweet spot that maximizes all of these goals. And that's what Faro is also uniquely suited to dig into the data and find that sweet spot that makes everyone happy.
Friederike von Waldenfels (19:47.953)
So is the product more like on a project base or is it something you do like a plug and play? you say, okay, I'm just using this Feral Labs, like say data warehouse with all the analysis behind I'm running my data through and then I get it out. Or do you need to integrate in existing systems? Like how is your product actually working?
Berk Birand (20:09.506)
Yeah, yeah, it's an excellent question. It's a software that runs continuously, and so the model that our customers engage in is that you they bring in the software and then run a pilot to show them how the software works and help them kind of set up their own first AI systems and AI models for specific use cases they may have in their factory and help them show them how to use the different tools in the software to troubleshoot problems and then get the answers faster and so forth.
After that time, we hand over the software to the engineers and they can use the software to receive the predictions and the recommendations, but they can also use it to build other models and other use cases that we might not even know about. They might not even want to tell us about. Maybe this is a side project that they think that if only we could model this particular aspect of the factory, maybe that would increase our yield and reduce our losses.
three months into the contract, could very well just take the data and have the Faroes AI systems have a go at it. And we believe that this model works really well because ultimately the experts, real domain experts are the engineers working in these factories. The consultancy model we find does not work very well. Actually, we have a, it was a funny anecdote from a couple of years ago, he was talking to a large German manufacturer that had hired a very large data science consultancy in Germany.
And they asked him to do some data nodes on their data set. then they sent the data. The consultancy worked on it for a couple of months. And then they came back with a report. And the report said, basically, you're manufacturing 20 types of products. And then, well, yeah, we know. We manufactured those products. We didn't need your three months in expensive payments to figure out how many products. So they had done some classification and so forth. So it's the.
Friederike von Waldenfels (21:50.375)
Yeah.
Berk Birand (22:02.83)
I think the interesting takeaway is that the people really that we want to empower are the engineers that understand the problems, that know what works and what doesn't work. And not just generally, but specifically to that one plant that they have most expertise in. And ultimately our goal is to provide the data infrastructure so that they can do their job better.
Friederike von Waldenfels (22:21.157)
And how does sustainability then play a part in this?
Berk Birand (22:24.782)
So the sustainability part is quite interesting in that the sweet spot that I mentioned around different goals. 20 years ago, sustainability would not be one of the parameters that would affect those different goals. There would be goals around cost cutting, profitability. It would be goals around yields, or maximizing the top line, quality. And it would try to find that middle ground that satisfies all of these in terms of the costs. Now there's an additional goal around sustainability.
that makes things even more complex than before. So now engineers have to deal with all the same problems plus the how do we combine the manufacture, the sustainability angle into this. So for example, in steel, we work a lot with electric arc furnace producers, so producers that melt scrap steel with electricity and then make it into new products, new steel products. And in those cases, the energy comes from the power plant. We have companies, for instance, one of our customers
is set up next to a hydropower plant. So their energy is completely essentially emission free, because they get it from hydropower. But they still have to buy ingredients, and their ingredients still have to be mined across the world. They have to be processed across the world, shipped across the world. So this has a substantial scope three contribution. So now these companies have to both navigate their cost structure in terms of how much we're paying and supply chains of these ingredients, but also have to factor in
How does the Scope 3 emissions come in? Do we want to buy products that are cheaper but have the higher Scope 3, which will start becoming a key component soon in terms of reporting? Or do we go, OK, we'll just pay more and reduce our cost basis for our and use a comparatively lower emission ingredient? So the emission component has made those competitions much, much more complex. And what we have done to adapt our software
is to add emission as a first-class citizen in terms of the optimizations. Previously, the optimizations involved around reducing the cost. So give us how much you're paying for one kilogram of your ingredients, how much you're paying for electricity, how much you're paying for running the factory for an hour and so forth. Now we have a whole other type of cost function revolving around the emission. So give us your scope one, two of your electricity consumption, scope three of your ingredients. And now we'll optimize both in terms of financial cost.
Berk Birand (24:48.18)
and environmental costs. And then we can give them a knob that allows them to tune it based on where they would like to be. This month, we want to favor financial costs. Next month, maybe we switch it the other way around. So being able to even have these conversations and being able to have these knobs to change, tune the way they operate their factory is just a completely new way of thinking about things.
Friederike von Waldenfels (25:15.911)
So coming now, you know, you're 10 years on the market and, know, 2015 probably sustainability, I don't know, you tell me is, was probably not on the top of the, of the agenda of the steel industry. And then came sustainability and now came AI and how do you see the evolvement is like sustainability still a big part or is it now AI driving it? I think you.
Berk Birand (25:26.326)
It was not, it was not even.
Friederike von Waldenfels (25:41.543)
You were always a little bit ahead of your time, probably we called machine learning before. So maybe you can tell us a little bit through, you know, like how, how did that evolve the public agenda and the perception? Whereas this would you have been offering? Like how, how could you benefit, but also, you know, saw the downside of it.
Berk Birand (26:04.142)
Yeah, absolutely. So, I mean, 10 years ago, sustainability was not one of the top priorities. It kind of goes in cycles, I think, you know, a couple of years ago, maybe five, six years ago, it started becoming more of a top of mind for companies, especially at the board level, I would say. I first at the board level, where a company would make commitments in terms of their 2030 goals. And I think at the time, the narrative was like, well, 2030 is way out there, you know, we have time, we'll figure things out until then, you know, who knows? Of course.
frequently leadership changes faster than these goals. So therefore, one set of leaders make commitments and then somebody else's problem in five, 10 years. So it was still a narrative, but it started becoming more more prominent. I think the interesting changes of the past five years, I would say, is first of all, the pandemic and of course, the supply chain shortages that came from it completely upended the entire way that factories and industrial sector was run.
assumptions that companies would make in terms of procuring ingredients in a certain timeline, in terms of kind of sources of ingredients, sources of ingredients, in terms of workforce, all became very much top of mind. So that was, think, one of the big changes and intersection of the digitization goals with sustainability and the pandemic, think, created an environment where people felt like, okay, well, we need to do something differently.
We can't just run our factories the way we've been running our factories for the past 30 years and expect to be around for the next 30 years. We need to adapt to the times. At the time, the fourth dimension of this, which is becoming more more prominent, is the labor shortages, in the industrial sector, which is, of course, more of an issue now and will become more more of an issue in the Western world specifically. Just for context, in Germany,
I believe that since the pandemic, half a million industrial manufacturing jobs have been lost. Part of it is, of course, the war in Ukraine, but part of it is also just a general trend of folks retiring, experienced people retiring, which is making it more more complicated for manufacturers in the Western world to run production in the Western world. So all of these combined created this kind of environment where we're now investing in digitization and data because we believe that's the right thing to do for...
Berk Birand (28:27.692)
securing the next 30 years of our production. We need to make sure we're much more aware that we can't rely on supply chains to be constant. Things change all the time and we need to adapt very quickly. Our labor problems are gonna get worse and more challenging in the next five, 10 years. And I think in this and the sustainability goals is the fourth one where we're now getting 2030 is not that far out anymore and we need to introduce things better in that context. And I believe AI kind of was uniquely suited
to help with all of these problems in some ways. And then again, finding a way to resolve problems on the labor shortage side by assisting engineers and making their jobs easier so that they can fix problems faster and ideally not have problems in the first place. This helps with the labor shortage side, the environmental side. Yeah, I think generally the last five years were absolutely instrumental for industrial layout. The next five years, I think are going to be even more instrumental when more and more of these systems will come online.
And of course, we'll continue to play a key role in that transition. actually, the interesting angle is that a lot of times when AI is discussed commonly, it's more around its impact on environmental goals because of the LLM specifically having such a large consumption, energy consumption impact. And of course, that is the case. And I have some opinions around how that might unfold in the next years.
We want always promote that there's AI systems that don't require that much energy that can run on a beefy workstation that can drive sustainability down. And that's where we want to place our bets in.
Friederike von Waldenfels (30:08.371)
Hmm. Interesting. Like imagine we are in the year 2050, where we all want to be net zero. And looking back, how does the world look like for you?
Berk Birand (30:15.608)
Heh heh.
Berk Birand (30:22.52)
Yeah, it's a great question. I think generally, I don't think we're going through the most optimistic of times. I think consumer confidence and know, there's a lot of things are pointing downwards, but I'm still hopeful. I'm very hopeful. I'm hopeful not because I think that magically, you know, humanity will come together and hold hands and make the right decisions that, you know, for the benefit of everyone, all of a sudden, I think that's a lofty goal. I hope we'll get there, but I'm not counting on it. But I think there's certain trends that are already in effect.
that are hard to stop. And I think there are certain market forces, including sustainability energy sources becoming cheaper than fossil fuel. In the US, as we all know, there's this drill baby drill, motivation top down. And many actually oil and gas companies are going, well, actually, we don't need to drill anymore. The prices are not where they, we don't need to. And I think that's going to accelerate more and more.
unless something major happens. And I do think there will be some breakthroughs on the nuclear side. mean, fusions, of course, would be great, and that's going to solve the problem. But even in terms of small reactors, in terms of reducing the regulation to make nuclear efficient, old school fission, more of a possibility. So I'm really hopeful that technology and science and these developments will actually get us to a kind net zero type of space. And of course, the AI
role of AI in this case will be to make sure that all of these infrastructures, infrastructure is running optimally. So when I think about 2050, I think of it as a time where I of like to picture this kind of solar punk view of, you know, green buildings and, you know, vegetation around them and then the world temperature is now back to, you know, below 1.5 degrees Celsius. And I think we can get there. Definitely we're working to have a minor contribution if we can to help us get there.
Friederike von Waldenfels (32:19.247)
Great picture. Let's just imagine at the top of it, like, you know, everyone is meditating and reading two hours of books every day. Because the second part, to be a little bit cynical, is what you said, you know, the labor shortage, what you describe right now in engineering, it's, you know, the AI is two-sided.
Berk Birand (32:24.59)
Exactly, that's yoga here.
Berk Birand (32:33.345)
Hmm.
Friederike von Waldenfels (32:41.953)
One is, you know, everyone says it's all going to steal our jobs at the same time. We actually experience a lot of labor shortage. Do you have an opinion about that?
Berk Birand (32:52.386)
Yeah, this is a topic that's near near to my heart. And this is actually even before starting Farrow, I interested in these topics, just getting into it. You know, what are we getting into here? we causing? I participating in the largest kind of, you know, change of the macro economic environment and the labor workforce? You know, it's funny at the time, you 10 years ago, they were there were narratives around, you know, truck drivers. That was kind of a big thing. Like, OK, autonomous driving is becoming a thing.
In the US, for example, there's like 3 million truck drivers employed. well, what was going happen to these 3 million truck drivers once we have autonomous driving, which is imminent, was the feeling of the time. The interesting thing, of course, is that 10 years later, truck drivers are fine. It's the engineers and software engineers that are more at risk, it seems like, that are more threatened by the new developments of the past two years. So around that time, 10 years ago, I was looking into the effects of automation. And of course, this is an area that's studied quite a bit.
It was studied quite a bit at the time, specifically in Germany, for example, there were research around the impact of robots in the automotive sector since the 70s and 80s. If the current AI systems is the fourth industrial revolution, the third industrial revolution was computerization of production and robots was a key part in it. And when looked at when those companies, academics that research in this, it's interesting that they saw the workforce actually going up
when robots came about as opposed to going down. And it was some jobs went down, know, welders, for example, let's say it's not a job that exists necessarily in automotive productions line, but there's not more people that maintain the robots. so overall, the industrial sector is doing better because they have more consistent, higher efficiency. There are more maintenance type of jobs and robot programmer type of jobs that didn't exist at the time. And as a result, you know, we came out of this with a...
Third Nus revolution on the positive side for labor generally. Now things are a little bit different now because now we're talking about AI in the sense that this is a more kind of cognitive, it's not just a physical kind of labor, but cognitive labor. There's a lot of talk around AGI coming in, super intelligence and so forth. I'm not personally sold that necessarily just because we had GPT in the last two, three years means that in three years we'll have AGI.
Berk Birand (35:15.558)
And you already see companies changing their tune to some degree, the large kind of AI companies. As we all know, the tech sector kind of thrives on making bold claims and raising a lot of money by saying that, things are around the corner. mean, Sam Altman last year said, know, AGI was a couple hundred days away. You know, he used that to raise a lot of money. And then now they release GPT 4.5, which was supposed to be GPT 5.
but it wasn't just good enough to call it five and therefore there would be the 4.5 and trying to change the tune. And we might very well have gotten to the kind of limits of where we can get with the current structure of LLMs. So I think there's this, always the, you know, if we extend the line, the curve, know, we'll get super intelligence in 10 years kind of narrative. I'm not convinced that that's going to be the case. I don't think that if we stick with the current technology, which is pretty good, I mean, it's quite incredible little things that we can do.
But there's still lot of hallucinations. There's still lot of issues with LLMs that mean, you know, it might improve efficiency, but is it going to completely erase jobs? I'm not so convinced. I mean, the manufacturing sector, you know, you need to make the right decisions 24-7, you know, around the clock every year. You know, it's unfeasible that an LLM can run a factory because it's unfeasible that 20 % of my production, LLM will say the wrong thing.
and I will fail my quality test potentially. I this is just not, it's not a possibility. And all of our models that we develop in the house, of course, are 99.999 % accurate, which is quite hard to do with models. So all in all, I do think there's gonna be changes in how the labor force is gonna be structured. I don't think it's gonna be a mass negative necessarily in the next five, 10 years, but 2050 is too far out. It could be that by the...
that there's another change in architecture of the LLMs that might drive an increase by 10, 15 years. And I think we need to prepare ourselves much so much by slowing down research, because I don't think that's really possible. I think it's funny always that the companies that are promoting slowing down research are the ones that are doing, like they want other people to stop doing research, which is, know, mean, it's perfect representation of how the tech people think.
Friederike von Waldenfels (37:35.783)
I think you cannot stop the curiosity of humans. It will be impossible. There will be always one person to be a little bit more expanding into a segment which is even more interesting, which is maybe, know, ethical, et cetera, on the edge of it.
Berk Birand (37:39.894)
Yeah, yeah, I think there's it's just
Friederike von Waldenfels (37:55.867)
Before we go into that discussion, like what you said, Elam, you use your own, so you developed your own model? just give like a one, two sentences about that. would be interesting.
Berk Birand (38:06.382)
Yeah, yeah, absolutely. Yeah, we develop our own models. LLMs of course work via text. Our models are models that work around understanding and approximating physical and chemical systems. So they work with numbers and specifically data coming from plants. So as a result, LLMs typically don't work as well in the kind of applications. So all of our models are time series, forecasting, modeling, and specifically time when you have not just one or two time series, but thousands. And all our models work on.
approximating and predicting these kinds of time series.
Friederike von Waldenfels (38:40.807)
Specifically, said like steel is one of your focus areas. Is there any other like two, three other major focus areas?
Berk Birand (38:48.406)
Absolutely. So, steel is one of our top areas. Chemicals would be the second. kind of within chemicals, different verticals, around CPG, special chemicals, commodity chemicals. And then cement would be our third area of focus.
Friederike von Waldenfels (39:02.031)
Okay. I think in one of the other interviews before, it was quite an interesting way of looking at it. It's like kind of the human decision-making, whereas the AI decision-making. And it's a little bit touching that what we discussed before. Can you maybe describe like one or two examples where you've seen, okay, the AI decision-making was actually way better.
But where's the human decision making? But where do we need human decision making in future to focus on to actually make the whole system better?
Berk Birand (39:40.28)
Yeah, ultimately, starting from the end, think the combination of human decision making and AI, so AI augmented human decision making is the part that is definitely the right way to do things today. This is an interesting subject because there are some manufacturers that don't trust AI to be able to connect to just purely for AI driven decision making. Others go, well, if we're to have humans anyway, this is not worth it. We need to have full AI decision making.
So I think I say the industrial sector, even it's split in terms of how to think about things. Everything that we do is human assisted decision making. So AI assisting humans on making decisions. And there's certain things that AI is very good at. It's very good at understanding, learning from a large set of data and understanding.
Friederike von Waldenfels (40:27.697)
Do you have some examples maybe like, you know, like make it.
Berk Birand (40:31.406)
Yeah, so for example, I mean, let's take a simple example since we've been considering a simple example. Let's say a steel company has been manufacturing a particular type of product, let's say making steel beams with a certain size for, you know, again, let's say construction example. And one construction site asked them to make it slightly differently, you know, make it a little bit thicker on the side and maybe the shape or the angle should be a little bit of an angle. And the plant may, the AI system has seen this type of production, a lot of production of this kind.
So then he could actually propose a way to do this in an efficient way. But if the ask is to devolve too far out from the comfort area, if they're saying, no, this is a completely new type of alloy that we're asking for, then it's very hard for AI to make recommendations because there's nothing enough in this dataset, in this training data. So because they haven't made steel of this kind. Whereas a human...
humans are much better generalizing and getting out of the comfort zone of the available data. And this is why AI is not as useful if you're starting a factory for the first time. Screen field deployment, I there's no data. I mean, how can you apply AI to it? So I believe the combination of AI doubling down on data that's in this comfort zone of previous production that has been run, combined with the human decision-making, which gets them to explore other parts
through domain knowledge and through expertise, that I think is absolute winner and that drives more value in fact as well.
Friederike von Waldenfels (42:05.959)
And I think the difference by Ferrolabs, like I think there is like a black box and white box AI, maybe you can say one or two words about that. Have you ever had a like an aha moment where an engineer or a factory leader went from like totally distrusting AI to fully embracing it?
Berk Birand (42:24.546)
Yeah, definitely. In fact, I would say it happens almost every time we do a demo of our software. The subtlety there is this, that this is an area that we've been promoting since almost day one. And namely, a lot of the AI models that are used in the enterprise world, not just in industry, but in retail or insurance and elsewhere, they revolve their AI models that are built by typically academia or large tech companies for their own needs.
So if you're a tech company and you're trying to forecast the load on your servers, for example, you don't need explanations. just need really good predictions. Or as another example, let's say you're building a computer vision model that will put an image and tells you what's on the image. I mean, you don't need to know why there's a cat on the image. mean, clearly, you would prefer to know for the model to tell you, think there's a cat. And this is why I think there's a cat. has ears and no.
But ultimately, you don't care. Just tell me what it is or which of my friends it is, and I don't need to know why. This is how most AI is built. Most AI models are built. This is how, know, chat GPTs and LMs are built as well, which is a huge problem. In fact, this is the reason why they have hallucinations is because they don't have explanations. If they had explanations or actual explanations, not just the reasoning type of model, then you would be able to figure out if they were hallucinating or not. So...
The type of AI models that we have developed, the issue that we have identified when starting Faro was that engineers don't want this. Engineers don't want some AI model that just tells you, here's what you should do without telling you why or without telling you how confident it is in the why. Engineers want their goal. In fact, the entire reason Six Sigma as a practice exists is to understand the root cause. you can only fix problems if you understand why they happen in the first place.
So a lot of our models are explainable. what that does, say, in the steel world is that an engineer can receive a recommendation and says, hey, add a little bit more carbon to this particular batch. And then, of course, the next question is, well, why? Well, because this particular scrap that you just melted, it has high levels of, let's say, chrome in its content. And as a result,
Berk Birand (44:44.066)
when you apply these kinds of rolling mill temperature pressures at the rolling mill, it's going to cause this. Therefore, you should apply, you should put a little bit less carbon on making this up. So this kind of explanation and combined with the confidence, every model will be wrong sometimes, even human models are wrong. But if models can convey how confident they are in their predictions, then engineers can actually make better decisions with that. They can go, okay.
Friederike von Waldenfels (44:59.919)
Mm.
Berk Birand (45:10.53)
The AI says, know, Faro's AI says do this and it's very confident, so I'll do it. And other times Faro's AI say, you know what, I'm not really sure, you know, cause I don't have enough data with this particular type of production. And so just fall back to what you've been doing, you know, before Faro's AI came about. And then the interesting thing is that when we go into conversations with industrial folks, this is always comes up as the central theme that they don't want to have a software that they don't understand that does not, that's not explainable running their factory for them.
For fear that if they rely on too much, then they'll actually lose track of things and things will start devolving slowly in the next years to the point where they won't be able to run the factory without the AI. And if the AI is wrong, they'll run the factory poorly.
Friederike von Waldenfels (45:56.45)
So coming to 2023, you raised, I think 15 million, right? As a last investment round. My curiosity kicks in. What has been more the reason to fundraise for investors, the sustainability part or the AI part?
Berk Birand (46:02.849)
Mm-hmm.
Berk Birand (46:14.926)
All of our investors from that round were climate tech investors. So definitely the sustainability part. think our investors also saw that AI will play a key role in driving emissions down worldwide. There's different approaches to sustainability. There's many hardware process-driven research that's happening, which is fantastic. Carbon capture is, of course, an example, but there's also examples around new types of steelmaking that is inherently less
wasteful or emits less. in whatever setup you use, whether you use electrolysis for hydrogen or carbon capture, doing things efficiently always improves your bottom line, basically. And as a result, our AI models, the thing that captured our investors' interests is that no matter which future direction we go through, if it's a hydrogen economy or if it's driven on carbon capture or about different trajectories towards net zero, if we keep wasting
resources while running the factories will make our job so much harder. And we estimate that actually factories worldwide making decisions slightly better using AI can drive 800 megatons of emission reduction worldwide from the industrial sector's emission budget. And the other part is that in the Western world, typically plants are more optimal and they emit less in the US specifically.
is doing great on the steel side in terms of emissions per ton of steel. But if we can't run factories in five, 10 years in the Western world because of labor shortages, then that's the worst case because then we're shipping production elsewhere in the world where they might not have as good of a trajectory in terms of their emission reduction goals. And I don't see how, even with the current labor shortages, I don't see how any manufacturer will be able to produce steel in the Western world in 10 years without the assistance of AI.
things are going to get harder and harder. So we do think it plays a key role in driving emissions down. And that's what got our climate tech investors really excited in addition to the AI.
Friederike von Waldenfels (48:19.303)
And what are going to use the money for? Like what are the big pockets you're going to use the money for? Like what are you going to focus on? What are your key priorities?
Berk Birand (48:28.206)
Yeah, so we're already working out of that round. And one of the big things that we've done in the last two years, of course, building up the team is ultimately always a goal with every round. I'm really excited about the team that we've built.
Friederike von Waldenfels (48:41.767)
How many people are you now?
Berk Birand (48:44.942)
We're at 23.
Friederike von Waldenfels (48:47.047)
Can you share some numbers? do you just close some numbers? Like how much revenue or anything like that?
Berk Birand (48:55.938)
We don't disclose revenue, but I can tell you that we have, in terms of the number of plants that we have on our system, around two dozen that are actively using. We have multiple AI models running for each of these plants around the clock, as we speak. So yeah, the team building and course expanding the go-to-market motion was a big goal for the round.
The other part that's been really, really exciting is to build a new product that we've released just six months ago or so that's been really, really successful so far, even though we just released it, around adding a whole other different way to help engineers troubleshoot problems easier and have essentially kind of an agent system that's running around the clock that continuously monitors the factory, understands when things are not right, and then runs a whole report.
that provides this report to the engineers so that they can understand why things went wrong. So we rolled this out six months ago and very successful so far and then lots of interest across all of our sectors of focus. And yeah, so that's been the trajectory.
Friederike von Waldenfels (50:03.015)
So soon raising again or is it gonna take a while?
Berk Birand (50:07.182)
Potentially, throughout this maybe Q4 or the next year probably would be the timelines that we're looking at. So far, we're really focused on executing and hitting our next milestones. So far, so good.
Friederike von Waldenfels (50:20.465)
Fantastic. So you are based in US, right?
Berk Birand (50:24.982)
I'm based in the US, so calling in from Brooklyn right now, but we have an office in Germany as well, in Düsseldorf in Germany. And we've always had a German-US DNA as well. Actually, one of the first things we've done since when we raised our seed round in 2017 was to start our Gmbh behind in Germany. So yeah, we're really excited about continuing to be a US-German company.
Friederike von Waldenfels (50:29.255)
Mm.
Friederike von Waldenfels (50:49.895)
How is that right now? Feeling like...
Berk Birand (50:53.452)
Yeah, it's definitely interesting times, interesting times for the German manufacturing sector, but lots of also positive positivity, you know, in terms of what's coming up. Ultimately, you know, one of things that we've learned with the German industrial sector is that, know, folks that are very, very resilient and they're very adaptive. They may not be the most agile necessarily, but ultimately, when they decide to take steps, they make really drastic steps and realign. And I'm seeing some signals of that.
these days. And I'm excited. I'm excited about the next. I think Germany will play a key role in continuing to be the bastion of production, industrial production of the Western world. I think it's unfortunate that we're going through a kind of protectionist era with respect to global manufacturing as well. But I do think ultimately we'll come out of this stronger and Germany will come out of it stronger as well.
Friederike von Waldenfels (51:45.095)
Interesting. my God, we could probably talk a lot more about that part too. Now it's such a damn that we're getting to the end, you know. Exactly, exactly. That would be actually amazing to talk about having this both sides sitting on both sides right now. I think it could be extremely interesting to talk about. But you know, like, unfortunately, we're coming slowly but surely to the end. Like looking back like 10 years is
Berk Birand (51:50.862)
Yes, let's block another three hours for that conversation.
Friederike von Waldenfels (52:14.755)
is a long time and it sounds like you are riding for a longer time on that as well, which I think is always great to hear to someone who's so passionate about what they're doing and actually want to build something longer than just seven years. I really enjoy that. So looking back at, you know, these 10 years has been like a, like a setback, which you really experience and think like, that was really tough. And what did you learn out of it?
Berk Birand (52:46.146)
Yeah, I think the probably the thing that was the an awakening was the realizing how hard it was to do enterprise sales as coming from a technical background and coming from academia. And I think we did a great job as technical founders, know, learn the ropes. And I think it is important for technical founders to understand what it entails and what challenges come about and then to be close, close contact with customers.
still of course try to be in close contact with customers. But that was a really moment of realization that, wow, this is really, really hard. There's so many moving parts and there's so much more appreciation for in general go-to-market and fee-call salespeople, including our sales team. It's really hard, man. It's really hard. So that was, I think, one of the things that was a first realization. And of course, the learning was that I think it's important that, again, the founders go through the sales period.
bringing on a professional sales team and marketing team early on would be probably the one thing that I would do differently and knowing what kind of profile to look for for these kinds of roles. again, are selling the industrial sector is very different to selling to data centers or other areas. And I think generally in the tech world, technical founders don't look very highly on sales folks and then go to market people. And I wasn't actually one of those people. But I think,
I think every engineer should try to sell something in an enterprise world specifically to understand what it entails. I think it's going to give them a whole new appreciation for the go-to-market people in their team.
Friederike von Waldenfels (54:25.287)
I always said, like I was in e-commerce before, everyone had to go through one day of customer service. And that totally, it was a different appreciation for that job. What kind of requests you get, what kind of question. Yeah, it's really, it gives really a good perspective on it.
Berk Birand (54:32.95)
Mmm, yeah. Yeah.
Berk Birand (54:41.134)
That's a great practice.
Friederike von Waldenfels (54:47.021)
Is there any like books or, know, podcasts or anything what you think significantly influenced you, like one of the AI side, but also on the sustainability side, which you could recommend?
Berk Birand (55:00.457)
Um, yeah, there's there's some, I think, you know, nothing comes to mind. I the main podcasts are generally great. And I think there's some great podcasters and including your podcast for the record. I think that I don't have any specific recommendations on books or podcasts. I think, yeah, generally, I try to listen to these, you know, on a weekly basis, you know, when I run or when I'm working out. And I think there's some some great podcasts out there. I can't think of anyone that would be more valuable than others.
Friederike von Waldenfels (55:29.959)
Thank you. Of course, sustain now is top of the list. If you could erase one misunderstanding about AI from the public mind, what would it be?
Berk Birand (55:33.614)
Exactly.
Berk Birand (55:44.47)
I would say it's very, so it would be the top thing would be this distinction between black box, white box, and that specifically that not all AI is necessarily black box. And I think maybe a close second would be to raise the notion that AI is a net consumer of energy or of emissions or creator of emissions, producer of emissions. And that's not necessarily doesn't have to be the case in their AI systems that are more
helpful for the emissions than hurtful.
Friederike von Waldenfels (56:15.975)
That's actually, I think, the takeaway what I usually ask as well at the end. So is that your one takeaway which listeners should take with them? Something to think about long after this interview?
Berk Birand (56:28.332)
I would think so. I think I'm generally excited about the advantage of AI. yeah, I think we should approach it with not take the hype at face value, but approach it still cautiously, but also optimistically. I think that's my takeaway as well.
Friederike von Waldenfels (56:48.761)
And coming now back to your beautiful painted picture of green buildings and everyone is in flowers. Amazing one. So what makes you confident that we actually can solve that climate crisis?
Berk Birand (57:04.206)
The part that makes me confident is the work of the science behind it and the fact that we have, despite the policy parts, which changes course really quickly, science doesn't change course that quickly. Funding of science changes course pretty quickly, unfortunately, which we're already seeing now, but the actual research itself doesn't. And there's always going to be people that will put their times and efforts and blood, sweat and tears into developing science and technology that will...
help us towards that goal. So that's the thing that I think is going to be the main driver for helping us get to that point by this utopia of 2050.
Friederike von Waldenfels (57:45.671)
Great. Okay. Thank you so much. I would have so many more questions in it. I think we definitely need to do a second round. Yeah, I really enjoyed that conversation. Thank you so much for joining this podcast. Is there any way how people can contact you?
Berk Birand (57:51.374)
I'll be very happy to.
Berk Birand (58:05.298)
They can go to our website and reach out. So ferrelives.com and we're a small team. So if they reach out, we would love to take any calls.
Friederike von Waldenfels (58:14.759)
Fantastic. So thank you so much for joining today.
Berk Birand (58:18.286)
Thank you so much for having me.
Comments